Mehdi Astaraki wins prestigious international challenge on image segmentation for radiation therapy
The rapid growth of deep learning-based methods has led to the development of a large number of unprecedented solutions for a variety of applications including in medicine. However, before the clinical application of such methods, their performance must be evaluated and validated extensively and objectively.
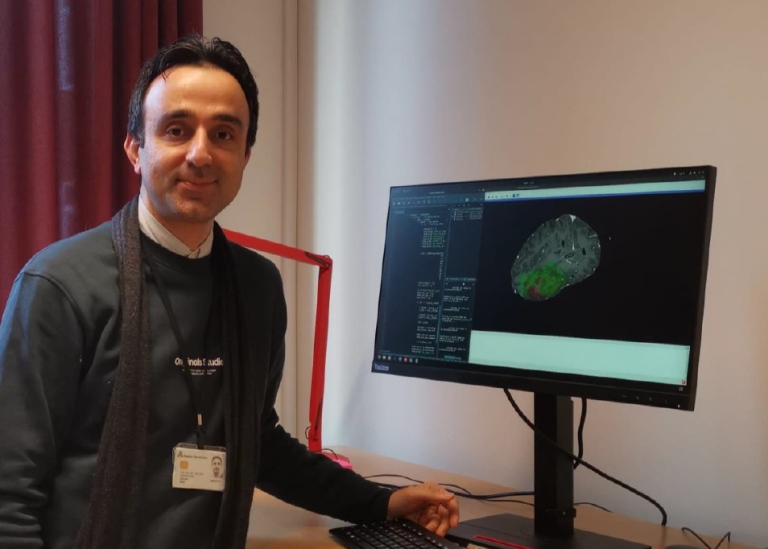
In recent years, international benchmarking competitions have become fundamental for the objective assessment and comparison of the developed methods. Mehdi Astaraki, postdoc in Medical Radiation Physics at Stockholm University, has recently won 1st place in a prestigeous competition organized by the International Conference on Medical Image Computing and Computer Assisted Intervention, (the most important and competitive community for medical image analysis tasks), for his deep learning-based method for tumour-, nodal-, and normal tissue segmentation in radiotherapy.
“I believe active contribution to the open source and open science communities is an inevitable part of research activities that can help us find better solutions”, says Mehdi.
Head and Neck (H&N) cancer is the seventh most common cancer globally accounting for more than 300,000 deaths per year, with radiation therapy as the standard treatment modality. The division of Medical Radiation Physics have several ongoing projects related to H&N cancer focusing on improving the quality of the treatment. A critical step in the radiotherapy chain is the meticulous definition of both tumoral regions and healthy structures based on Computed Tomography (CT) images.
Therefore, the development of a robust computerized method to automatically detect and segment the tumors and healthy tissues can both speed up and improve the treatment planning process. Mehdi has developed a deep learning-based method to precisely segment tumoral regions, affected lymph nodes, and surrounding healthy structures in CT images of the H&N region.
To evaluate and validate the method, he participated in the Segmentation of Organs-at-Risk and Gross Tumor Volume of Nasopharyngeal Carcinoma for Radiotherapy Planning (SegRap2023) challenge, a competition organized by the International Conference on Medical Image Computing and Computer Assisted Intervention, MICCAI. MICCAI is the most important and competitive community for medical image analysis tasks, and the annual conference attracts the world’s leading biomedical scientists, engineers, and clinicians from a wide range of disciplines associated with medical imaging and computer-assisted intervention.
The goal of the SegRap2023 challenge was to develop automatic tools to segment 54 healthy structures (Task 1) and 2 tumoral regions (Task 2). The challenge was held in three phases: in phase 1) organizers released only 120 image data for participants to develop their algorithms locally; in phase 2) participants should submit their algorithms to be evaluated on 20 subjects; and in phase 3) final evaluation with submitted algorithms on 60 subjects.
A total of 395 teams from around the world registered for the challenge with 230 teams from China, and 32 teams from the US as the highest participant countries. In the second phase, each team could submit up to 10 different algorithms, however, in the last phase, each team was allowed to submit only one single algorithm.
In the second phase, more than 150 models were submitted for Task 1 and more than 70 models for Task 2. For the final phase, more than 30 models were evaluated for each task as well. Participants in the challenge included several leading research groups as well as teams from well-recognized imaging companies including Canon.
Mehdi’s contribution won 1st place in the final evaluation phase for Task 2 and won 2nd place in the second evaluation phase for both tasks. The details of the methods were presented on the 12th of October at the MICCAI conference held in Vancouver, Canada where the team from Stockholm University was announced as the winners of the competition.
The next step for Mehdi and his collaborators is to incorporate the model with the research PACS, a Picture Archiving and Communication System used mainly in healthcare to securely store communicate medical images.
“By deploying the developed model on the research
PACS of the hospital, the performance of the model can be validated against clinical datasets for the subsequent application in radiation treatment planning”, Mehdi says.
Mehdi will present his work in a News and Views session during spring, details to be announced later.
Last updated: December 12, 2023
Source: Fysikum