Machine Learning and the Stock Market
An article by Abalfazl Zareei (SBS) and Jonathan Brogaard (University of Utah) has been accepted for publication in the Journal of Financial and Quantitative Analysis, one of the top journals in Finance.
The article, entitled Machine Learning and the Stock Market, addresses the dispute between academia and investment professionals on the usefulness of technical analysis.
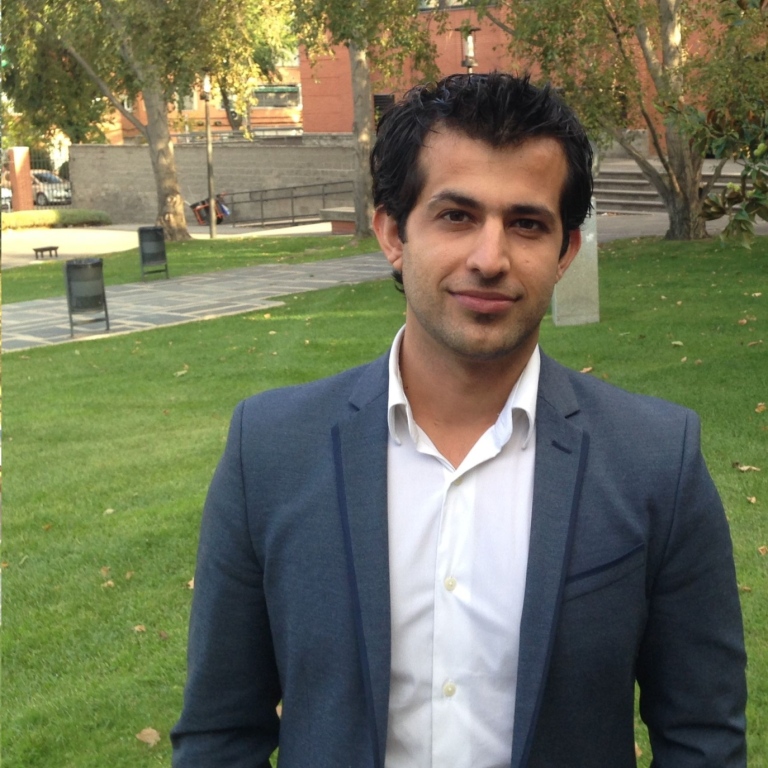
Recent academic studies show that technical analysis does not add value to the investment process for two reasons: First, it is not possible to find the profitable trading rule ex-ante, and second, the profitability of technical trading disappears as soon as the costs of trading are considered.
The authors investigate the usefulness of technical analysis by using machine learning technology. In other words, they asked the machines to pick the technical trading rule for future trading. The results show that the machines are successful in selecting the profitable trading rules ex-ante.
However, this profitability decreases over time, coinciding with the rise in computational power and indicating an increase in market efficiency.
Last updated: January 28, 2022
Source: SBS