AI has a bright future in medicine
AI technology is breaking new ground in all areas, not least in medicine. What can we expect in a near and distant future? PhD students and supervisors from five countries gathered at Stockholm University to discuss their projects and learn from each other.
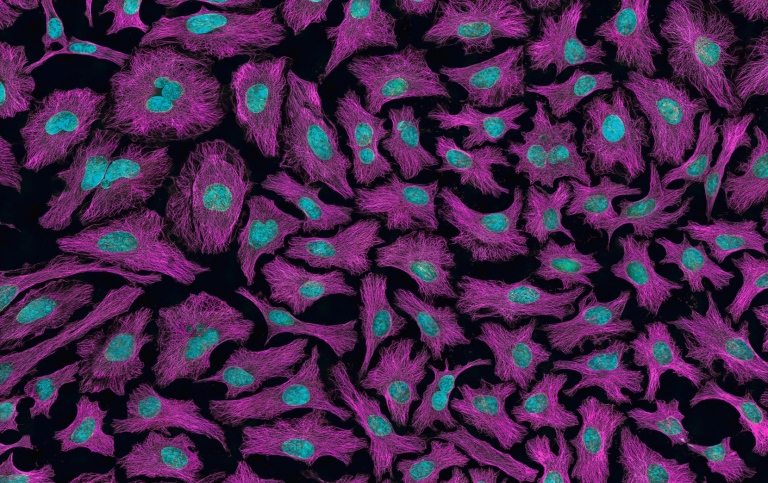
“We’ve had a great couple of days. It’s been very interesting to hear the presentations and talk to PhD students from Italy, Portugal, Germany, UK, and – of course – Sweden. I think we all learned a lot”, says professor Panagiotis Papapetrou, Department of Computer and Systems Sciences (DSV) at Stockholm University.
He hosted the 2nd European Medical Artificial Intelligence (MedAI) PhD School on September 12–13, 2023. The first day was organized at DSV in Kista, and day two was spent at sea – on a boat to Finland.
25 European participants, all deeply involved in the growing stream of research that combines artificial intelligence with medicine and health, shared insights from their projects.
There was also a keynote speech from professor John Holmes, University of Pennsylvania, USA, who joined the workshop for both days. The topic of his talk was “Explainability, Bias, and Fairness in Machine Learning: Threats to the integrity of inferences made from data using machine learning”.
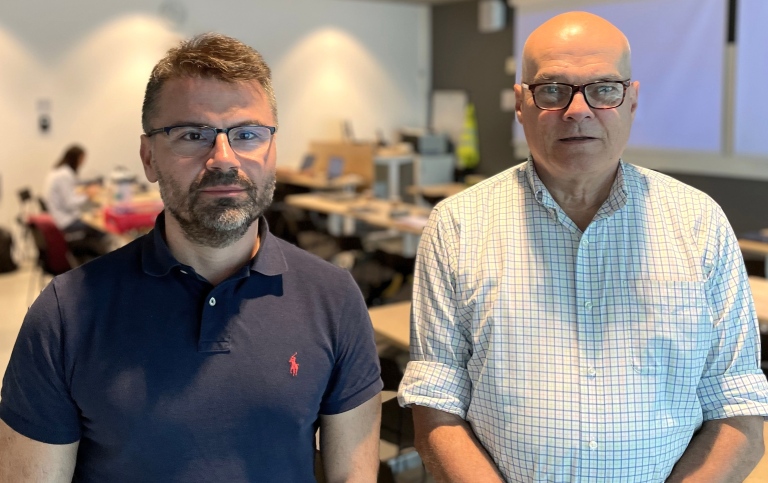
Explainable AI is challenging
Explainable AI is a key concept for this group. It has to do with the AI’s ability to explain how and why it reaches a certain conclusion. If, for example, a medical doctor should be able to trust the medication that the AI suggests, the AI have to be able to explain its recommendation.
“Explainable AI is a must in medical applications, and there are at least four challenges for explainable AI in medicine: interpretability, understandability, usability and usefulness. Deep analysis of the problem domain and users is required”, says Panagiotis Papapetrou.
“Another important takeaway from the workshop is that we always have to describe our data and its characteristics when applying machine learning in medical datasets. This makes our results meaningful and trustworthy”, he continues.
Important meetup for students and mentors
During the COVID-19 pandemic, Papapetrou realized that his PhD students were missing out on the important networking that is usually done at international research conferences. He teamed up with some colleagues at other universities and a first online version of MedAI was held in 2020.
Next year, we are planning to invite one more partner
So far, two online events and two physical events have been organized – and the plan is to continue this effort.
“We consider it very important for PhD students to get to know what other research groups in Europe are working on. At MedAI, they receive feedback from mentors that are senior in the area, while meeting other PhD students and initiating possible collaborations.”
Growing network
At the moment, five university partners are involved in the collaboration: Stockholm University, Brunel University in the United Kingdom, University of Magdeburg in Germany, University of Porto in Portugal and University of Pavia in Italy.
“We all have our different research interests, but a common theme among us is how to handle multimodal data when building AI models for medical applications. Next year, we are planning to invite one more partner and we hope to organize the workshop in Italy. We are also investigating the possibility of acquiring funding for building a Marie Curie PhD student consortium in Europe within the area of Medical AI”, says Panagiotis Papapetrou.
More about the research
The five university partners – Brunel University, University of Magdeburg, University of Porto, University of Pavia, and Stockholm University – all focus on different research questions.
– Brunel Team: Focusing on data quality, privacy and utility. How can we generate synthetic medical data that mimic real ones, and how can it be shared safely without privacy concerns?
– Magdeburg Team: How can we handle irregular data and develop personalized predictions? How can we find interesting and surprising patient subgroups?
– Pavia Team: How should we handle multimodal data? How do we assess the quality and reliability of predictions in the medical context?
– Porto Team: Medical focus. How to perform patient triage in the emergency unit? How to bridge the gap between machine learning and clinical practice?
– Stockholm Team: AI model explainability, multimodal learning (i.e. building machine learning models that exploit different data modalities and their interactions). Much of the research is carried out within the Data Science Research Group.
Text: Åse Karlén
Last updated: September 19, 2023
Source: Department of Computer and Systems Sciences, DSV