Are doctors ready for AI assistants?
AI in healthcare is a promising – and challenging – field. Analysing massive amounts of multimodal patient data could result in better treatments. But first, medical doctors need to trust the models.
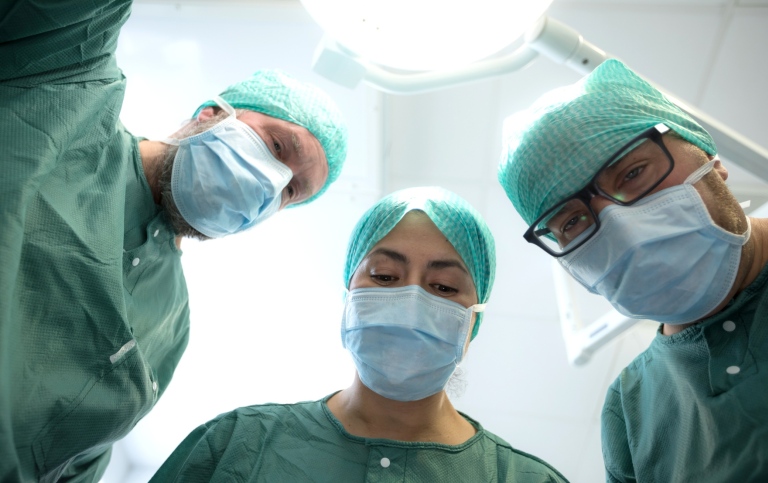
On February 6, 2024, the Department of Computer and Systems Sciences hosted a Tech Tuesday event for the tech community in Kista. Professor Panagiotis Papapetrou was the speaker, sharing interesting insights from his research on explainable AI for healthcare.
“Healthcare is a challenging domain. We want our algorithms to be as well performing as possible, but sometimes we ignore an important factor: Trust,” said Panagiotis Papapetrou.
This is where explainability comes into play.
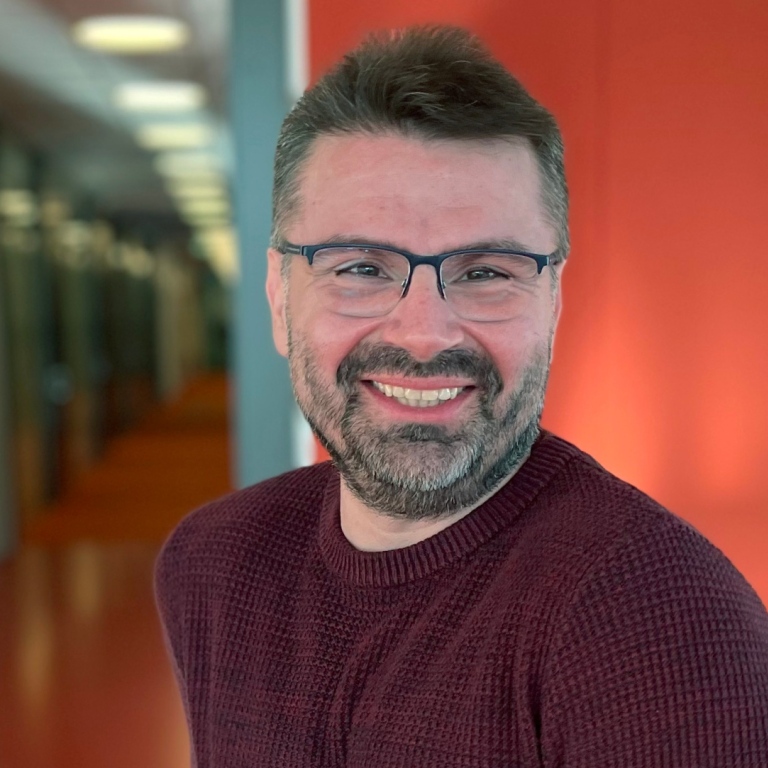
“Our experts – in this case, the doctors – have to understand why a model has come up with a decision. To gain the doctor’s trust, AI has to explain what lies behind its recommendation.”
Actionability is another important ingredient, according to Papapetrou. Recommendations given by an AI must also be possible to act upon. Not until then will AI models be able to improve healthcare.
Massive and complex data
But several challenges have to be overcome before trustworthy and actionable AI models can start to assist human doctors in full scale. Protecting the privacy of patients and avoiding bias are two such challenges.
Another challenge has to do with the data that is being fed into the models.
“Healthcare data is massive and complex. It is generated from many different sources,” said Panagiotis Papapetrou.
In this challenge lies also the big opportunity: When massive and complex data is analysed, we can find answers to complicated problems.
The brain of AI is machine learning
Given that a single patient produces more than 80 megabytes of medical data every year, the datasets tend to be huge. Notes from nurses and doctors, lists of medicines, medical imaging such as MR scans and X-rays, data from wearables that trace movement, heart rate and sleeping cycles, results from clinical trials, and genome registries are just some of the different types of medical data that are piling up.
“We see many opportunities for data-driven decision making – and a need for AI and machine learning.”
“The brain of AI is machine learning. It helps us identify and recognise patterns,” Papapetrou explained.
Humans are still in charge
He made it clear that introducing advanced AI tools in health care does not mean that human clinicians will be replaced.
“AI can search through billions of similar cases and suggest what tests the patient should take, or which combination of medications they should try. And in surgery, which requires precision, a pair of steady robot hands can help. The important thing is that the doctor or medical expert always takes the final decision. He or she has full responsibility,” said Panagiotis Papapetrou.
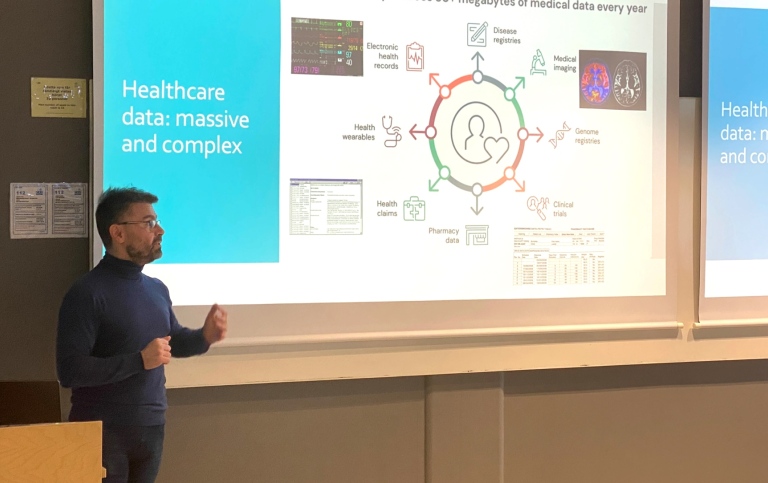
About the event
Tech Tuesday is a monthly networking and knowledge-sharing event for the tech community in Kista. It is organised by Kista Science City.
On February 6, 2024, the Department of Computer and Systems Sciences (DSV) at Stockholm University was the host of the event.
Professor Panagiotis Papapetrou, DSV, was the speaker. The topic was “Explainable AI for healthcare – challenges and future outlook”.
The event was fully booked early on, and there was a waiting list. Almost 50 participants from big tech companies, small startups, academic institutions and public agencies joined the seminar at DSV.
More information on DSV’s research in this area
Text: Åse Karlén
Last updated: February 20, 2024
Source: Department of Computer and Systems Sciences, DSV