Research project MS2Tox: Deep Learning for Automated Prediction of the Endocrine Disruptive Potency of Chemicals
MS2Tox: Deep Learning for Automated Prediction of the Endocrine Disruptive Potency of Chemicals in Complex Mixtures
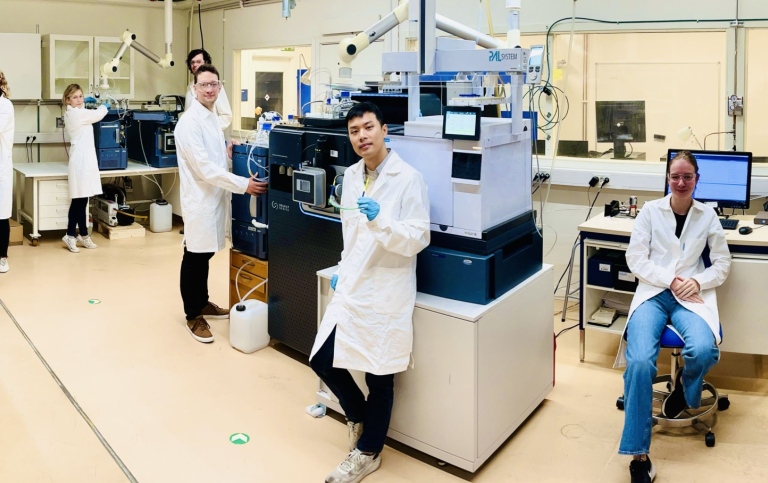
The safety of water, food, and new materials used in daily life is essential for human life and the ecosystem. Nontarget liquid chromatography high resolution mass spectrometry (LC/HRMS) is increasingly used to detect chemicals in such samples. Here we will develop machine learning methods for, evaluating the hazard possessed by the chemicals in these complex mixtures, especially endocrine disruptive potency. Up to now the main limitation in applying machine learning has been that for predicting toxic endpoints of complex mixtures the single chemical constituents in the mixture need to be first unequivocally identified. Here we will predicting the hazard of chemicals directly from the empirical spectral information acquired rapidly in nontarget LC/HRMS chemical analysis.
Project members
Project managers
Anneli Kruve
Associate Professor
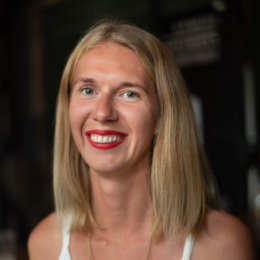
Jonathan Martin
Professor
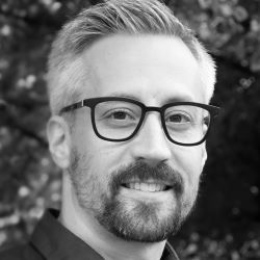
Members
Yvonne Kreutzer
PhD student
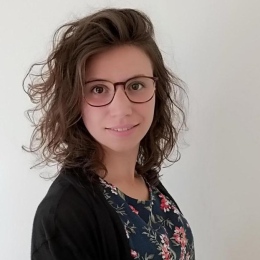