Disputation: Mahbub Ul Alam
Disputation
Datum: torsdag 28 mars 2024
Tid: 13.00 – 17.00
Plats: Lilla hörsalen, DSV, Borgarfjordsgatan 12, Kista
Välkommen till en disputation på DSV! Mahbub Ul Alam presenterar sin avhandling som handlar om hur maskininlärning kan bidra till en mer effektiv vård. Hans forskning kretsar kring Covid-19 och sepsis.
28 mars 2024 presenterar Mahbub Ul Alam sin doktorsavhandling på Institutionen för data- och systemvetenskap (DSV) vid Stockholms universitet. Titeln är ”Advancing Clinical Decision Support Using Machine Learning & the Internet of Medical Things: Enhancing COVID-19 & Early Sepsis Detection”.
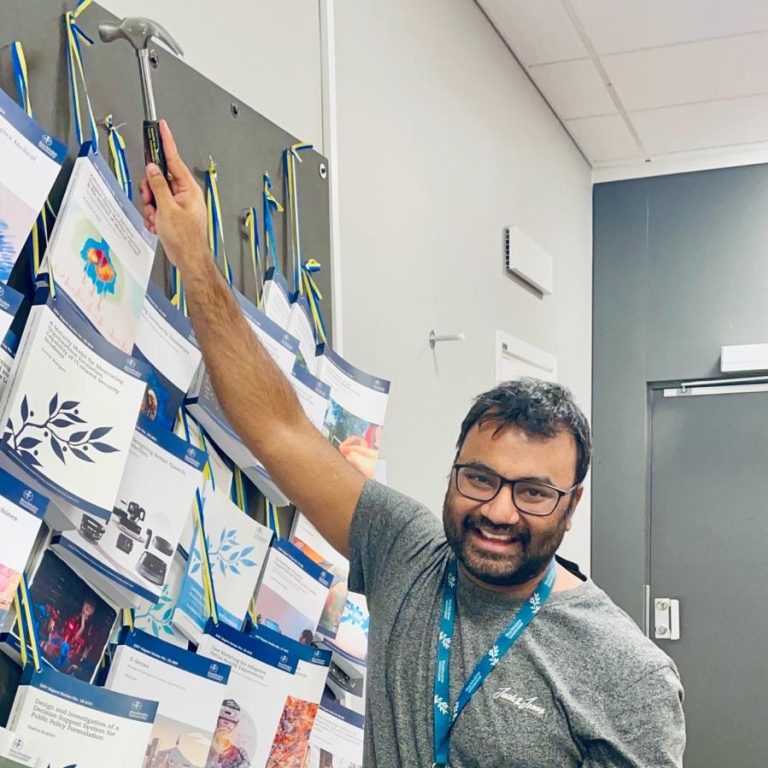
Doktorand: Mahbub Ul Alam, DSV
Opponent: Sadok Ben Yahia, University of Southern Denmark
Huvudhandledare: Rahim Rahmani, DSV
Handledare: Jaakko Hollmén, DSV
Ladda ner avhandlingen från Diva
Kontaktuppgifter till Mahbub Ul Alam
Disputationen genomförs i DSVs lokaler i Kista, med start klockan 13.00.
Hitta till DSV
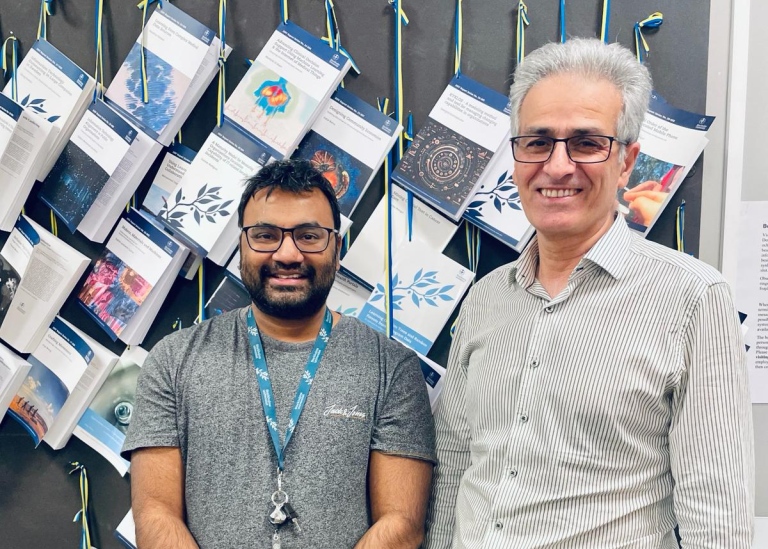
Sammanfattning (på engelska)
This thesis presents a critical examination of the positive impact of Machine Learning (ML) and the Internet of Medical Things (IoMT) for advancing the Clinical Decision Support System (CDSS) in the context of COVID-19 and early sepsis detection.
It emphasizes the transition towards patient-centric healthcare systems, which necessitate personalized and participatory care—a transition that could be facilitated by these emerging fields. The thesis accentuates how IoMT could serve as a robust platform for data aggregation, analysis, and transmission, which could empower healthcare providers to deliver more effective care. The COVID-19 pandemic has particularly stressed the importance of such patient-centric systems for remote patient monitoring and disease management.
The integration of ML-driven CDSSs with IoMT is viewed as an extremely important step in healthcare systems that could offer real-time decision-making support and enhance patient health outcomes. The thesis investigates ML's capability to analyze complex medical datasets, identify patterns and correlations, and adapt to changing conditions, thereby enhancing its predictive capabilities. It specifically focuses on the development of IoMT-based CDSSs for COVID-19 and early sepsis detection, using advanced ML methods and medical data.
Key issues addressed cover data annotation scarcity, data sparsity, and data heterogeneity, along with the aspects of security, privacy, and accessibility. The thesis also intends to enhance the interpretability of ML prediction model-based CDSSs. Ethical considerations are prioritized to ensure adherence to the highest standards.
The thesis demonstrates the potential and efficacy of combining ML with IoMT to enhance CDSSs by emphasizing the importance of model interpretability, system compatibility, and the integration of multimodal medical data for an effective CDSS.
Overall, this thesis makes a significant contribution to the fields of ML and IoMT in healthcare, featuring their combined potential to enhance CDSSs, particularly in the areas of COVID-19 and early sepsis detection.
The thesis hopes to enhance understanding among medical stakeholders and acknowledges the need for continuous development in this sector.
Nyckelord
Internet of Medical Things, Patient-Centric Healthcare, Clinical Decision Support System, Predictive Modeling in Healthcare, Health Informatics, Healthcare analytics, COVID-19, Sepsis, COVID-19 Detection, Early Sepsis Detection, Lung Segmentation Detection, Medical Data Annotation Scarcity, Medical Data Sparsity, Medical Data Heterogeneity, Medical Data Security & Privacy, Practical Usability Enhancement, Low-End Device Adaptability, Medical Significance, Interpretability, Visualization, LIME, SHAP, Grad-CAM, LRP, Electronic Health Records, Thermal Image, Tabular Medical Data, Chest X-ray, Machine Learning, Deep Learning, Federated Learning, Semi-Supervised Machine Learning, Multi-Task Learning, Transfer Learning, Multi-Modality, Natural Language Processing, ClinicalBERT, GAN
Senast uppdaterad: 7 mars 2024
Sidansvarig: Institutionen för data- och systemvetenskap, DSV