Disputation: Zhendong Wang
Disputation
Datum: onsdag 4 december 2024
Tid: 09.00 – 12.00
Plats: Aula Nod, DSV, Borgarfjordsgatan 12, Kista
Välkommen till en disputation på DSV! Zhendong Wangs doktorsavhandling handlar om hur maskininlärningsmodeller kan förbättras och användas exempelvis inom sjukvården.
4 december 2024 presenterar Zhendong Wang sin doktorsavhandling på Institutionen för data- och systemvetenskap (DSV) vid Stockholms universitet. Titeln är ”Constrained Counterfactual Explanations for Temporal Data”.
Doktorand: Zhendong Wang, DSV
Opponent: Mykola Pechenizkiy, Eindhoven University of Technology, Nederländerna
Huvudhandledare: Panagiotis Papapetrou, DSV
Handledare: Isak Samsten, DSV
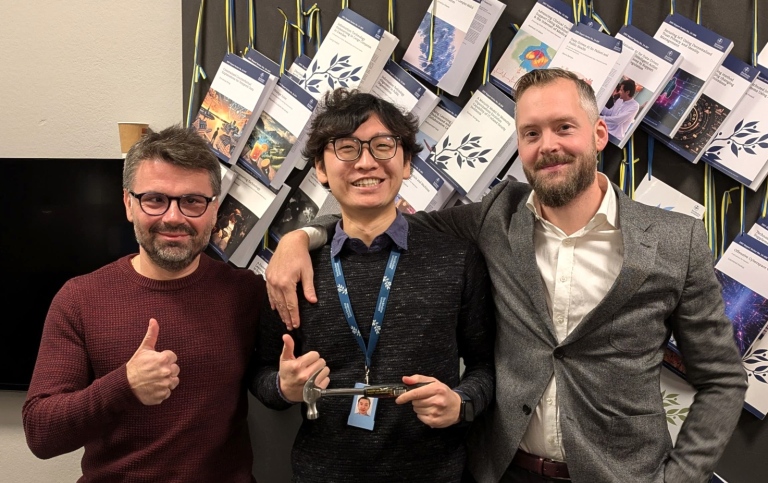
Ladda ner avhandlingen från Diva
Kontaktuppgifter till Zhendong Wang
Disputationen genomförs i DSVs lokaler i Kista, med start klockan 9.00.
Hitta till DSV
Sammanfattning på engelska
Recent advancements in machine learning models for temporal data have demonstrated high performance in predictive tasks like time series prediction and event sequence classification, yet these models often remain opaque. Counterfactual explanations offer actionable insights into these opaque models by suggesting input modifications to achieve desired predictive outcomes. In the context of explainable machine learning methods, there is a challenge in applying counterfactual explanation techniques to temporal data, as most previous research has focused on image or tabular data classification. Moreover, there is a growing need to extend counterfactual constraints to critical domains like healthcare, where it is crucial to incorporate clinical considerations.
To address these challenges, this thesis proposes novel machine learning models to generate counterfactual explanations for temporal data prediction, together with incorporating additional counterfactual constraints. In particular, this thesis focuses on three types of predictive models: (1) event sequence classification, (2) time series classification, and (3) time series forecasting. Furthermore, the integration of local temporal constraints and domain-specific constraints is proposed to emphasize the importance of temporal features and the relevance of application domains through extensive experimentation.
This thesis is organized into three parts. The first part presents a counterfactual explanation method for medical event sequences, using style-transfer techniques and incorporating additional medical knowledge in modelling. The second part of the thesis focuses on univariate time series classification, proposing a novel solution that utilizes either latent representations or feature space perturbations, additionally incorporating temporal constraints to guide the counterfactual generation. The third part introduces the problem of counterfactual explanations for time series forecasting, proposes a gradient-based method, and extends to integrate domain-specific constraints for diabetes patients. The conclusion of this thesis summarizes the empirical findings and discusses future directions for applying counterfactual methods in real-world scenarios.
Nyckelord
Counterfactual explanations, Deep learning, Explainable machine learning, Healthcare
Senast uppdaterad: 21 november 2024
Sidansvarig: Institutionen för data- och systemvetenskap, DSV