Keenious – An AI Search Engine
Keenious is an academic search engine that recommends research papers by analysing documents using artificial intelligence algorithms. You can use Keenious both in your web browser and in Microsoft Word or Google Docs.
What is Keenious?
Keenious is a recommendation tool for academic articles where you search for similar or related articles based on either an article or your own written text. Instead of searching using individual search terms, Keenious analyses texts using AI and presents search results based on the topic of the text.
The articles in Keenious comes from a curated subset of OpenAlex. OpenAlex is an open-source catalogue with both open-access and paywalled journal articles, conference papers and workshop papers. The Keenious subset only includes journal articles and preprints and has excluded articles lacking essential information such as for instance author’s name, date of publication or valid DOI.
Keeniuos can analyse texts in many languages, including Swedish. Non-English texts are translated to English by Keenious (using Microsoft Azure's Translator Service). The translation is used as input retrieving research literature in English. For results in the same non-English language as in your document you uncheck the 'Cross Language' option in the upper-right corner. Note that there is a limitation of 20,000 characters (3000-5000 words) for text translations. There is no such limit for texts in English.
Register an account
Keenious is available to all students and staff at Stockholm University. Register a Keenious account with your SU e-mail address in order to use all Keenious’ features and to be able to access articles available through library subscriptions. For students the email address is the SU username followed by @student.su.se.
How to use Keenious
Keenious is a different search tool than you might be used to. Here you use a document as an input into the search rather than search terms. In order to get the best results, there are five tools that are important to know about. These include topics, focus search, similar articles, filter by keywords and filter by publication date, citation count, or open access (OA).
The video gives you an introduction to how to search with Keenious and use these tools both in your web browser and in Microsoft Word.
Read more about how to use Keenious on Keenious Help pages
Use Keenious in your web browser
Keenious can be used directly in your web browser. You can log in with your personal account at Keenious’ website and either paste the text you want to analyse or either drag and drop or upload a PDF.
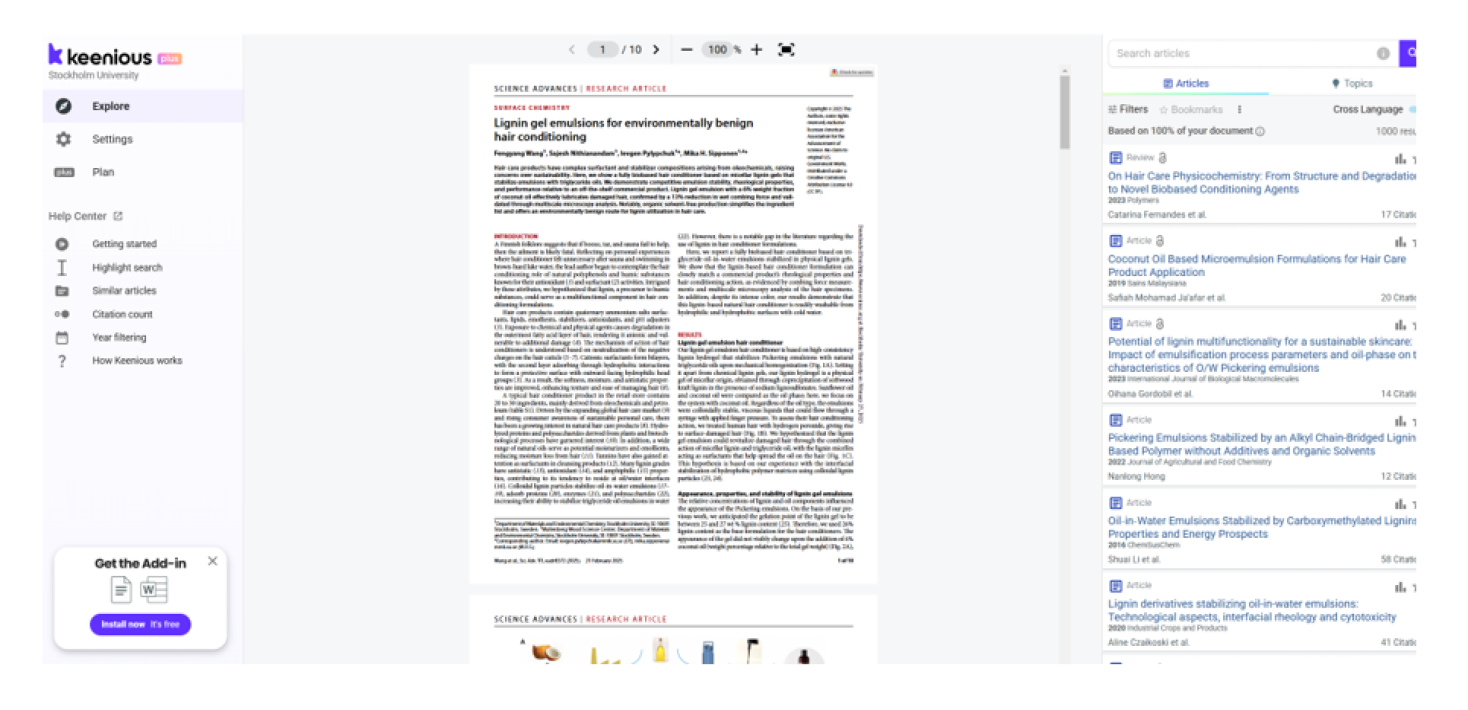
Go to Keenious in your web browser
Use Keenious in Microsoft Word and Google Docs
Keenious is also available as an addon for Microsoft Word and Google Docs. It appears as a sidebar next to your text and analyses your text and gives recommendations of articles related to your written text.
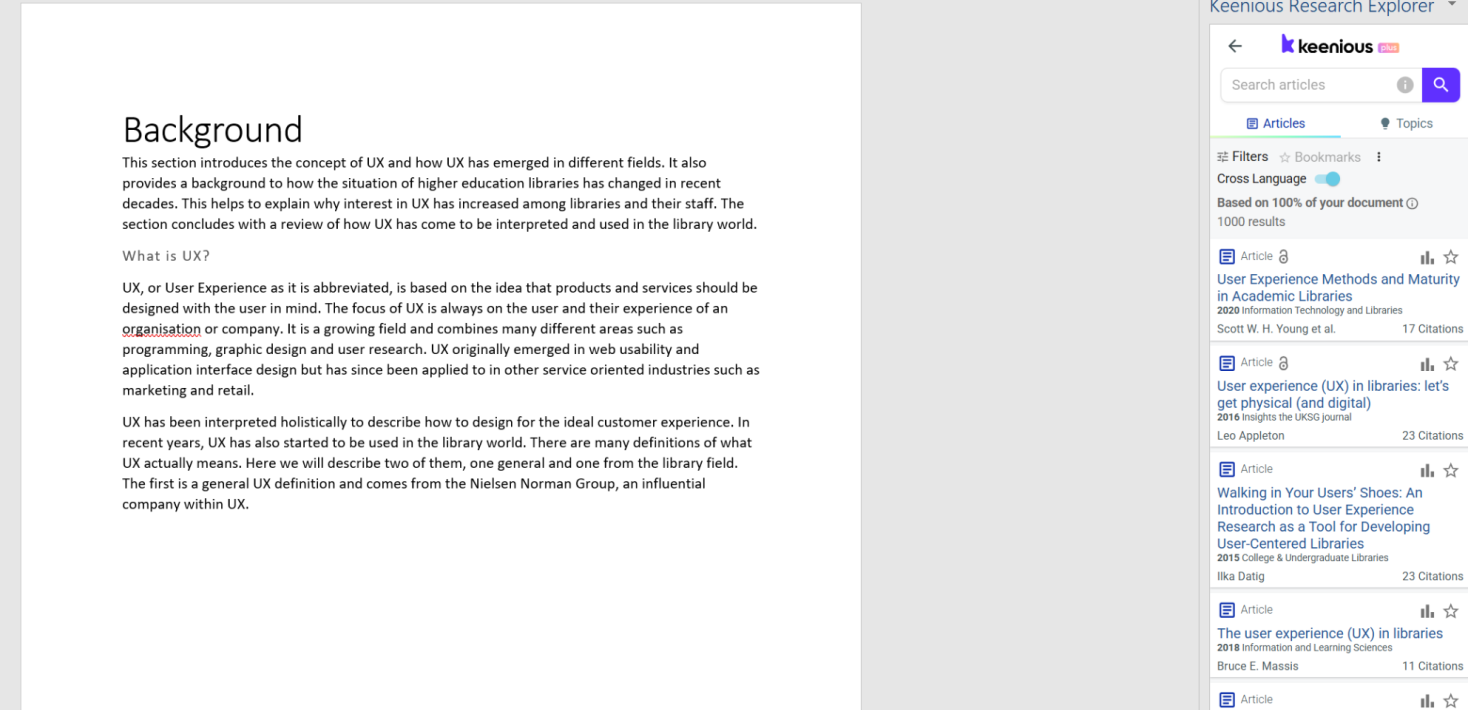
Download Keenious for your word processor
Why use Keenious?
Since Keenious does not rely on search terms it can be useful if you have difficulty searching in traditional databases. It is also useful if you want to extend your search beyond those. However, you should not treat Keenious as an alternative but rather an addition to searching in databases or the library search service.
Keenious is useful at least for the following purposes:
- Find ideas and learn new concepts. If you are new to a subject or unsure of what you want to write about searching in traditional databases can be challenging. Here Keenious can be a useful way to help find ideas to get started with an essay and learn new concepts.
- Find similar and related articles. If you have found some useful sources already you can use Keenious to help you find related and similar articles.
- Search as you write. With the addon in Microsoft Word or Google Docs you can search for relevant articles while you are writing by using the Keenious sidebar. You can also highlight specific parts of your text to find relevant articles to that part of your text.
- Extend your search and promote an interdisciplinary approach. Since Keenious has a huge scope, it can help you find articles that might not be included in the databases you normally search, especially if they are more subjectsubject specific databases.
- Identify key research and researchers in a field. The number of times an article has been cited can be useful as a measure of an article's authority and importance within its field. Since Keenious rewards articles with many citations and also allows you to filter by citation count you can use it to find highly cited articles. However, articles can be cited frequently for other reasons than their academic merit. Also, citation count is affected by publication date, the prestige of the journal, and citation practices within a particular field.
When not to use Keenious
- If transparency and replicability is key to your method Keenious is not suitable. Since Keenious uses an AI algorithm it is not possibly to explain exactly how Keenious searches. It is therefore not possible to search systematically in Keenious. Traditional academic databases give you much more control over your search and the possibility to document it.
- If you want to make an exhaustive search. Keenious is not designed for exhaustive searches where you aim to find all available research for a delimited research question. If your aim is to conduct an exhaustive search you should instead use traditional academic databases.
How Keenious rank their recommendations
Keenious uses many different factors to predict and make recommendations. However, to simplify, there are four key factors that influence the ranking.
- Shared terms: Keenious takes into account the number of shared rare terms that are found in your text and in the titles and abstracts of the articles it recommends.
- Predicted meaning of your text: Keenious uses a language model to predict the meaning and topic of your text. This makes it possible to find articles that do not share many rare terms but are still relevant. It also helps prevent including articles that do share many rare terms but are still not relevant to you.
- Citation count: Keenious considers how many times an article has been cited in other articles ranking those with a high citation count higher.
- Publication date: More recent articles are given precedence.
Keenious and systematic reviews
Since it is not possible to make the type of exhaustive database searches required in systematic reviews Keenious is not suitable for that part of the review process. However, it can still be useful at some parts of the process.
- Preliminary searches. Keenious can help you explore existing literature on the subject in the early stages of the process helping you examine the originality of your research question. Since Keenious analyses texts you can include detailed descriptions of your subject to examine if there are similar articles already published.
- Formulating the search strategy. An essential part of forming a search strategy for a systematic review is to find all relevant search terms. Using Keenious can help you find alternative keywords and synonyms to add to your search strategy.
- Additional searches. After you have screened the articles retrieved in your search in traditional academic databases you can transfer them to Keenious to see if Keenious might suggest articles that your database search potentially missed.
Keenious support
You can find more instructions and answers to frequently asked question on Keenious’ website.
You can also contact Keenious on their website if you have questions
Last updated: March 4, 2025
Source: Stockholm University Library